Overview
My research aim is to answer fundamental questions about the structure of human knowledge. We learn by interacting with our environment, and what we learn depends therefore on both our surroundings and our mental capacities. How should we conceive of this relationship? How can we quantify, or formalize, the contributions of evidence from the environment and the filter of prior expectation? What things are readily learned, and once learned, readily communicated? My approach to these questions is to mix computational modeling with behavioral experiments in the context of basic cognitive skills. From the way we learn how to catch a fly ball, or to tell ripe fruit from rotten, to the way we decide which apartment to rent or what car to buy, cognitive skills require the careful education of attention to some of the myriad sources of information the world provides. My goal remains to provide an account of the nature of the mechanisms that enable us to exercise these skills.
Solving Plato’s Other Problem
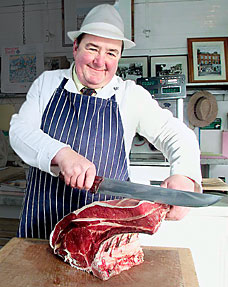
“… to be able to cut up each kind according to its species along its natural joints, and to try not to splinter any part, as a bad butcher might do.”
Early in my career I set out to study category learning, and, starting with my postdoctoral work, I have been pursing an exemplar-based approach to categorization ever since. This approach uses an analogy of storage and retrieval of instances to describe the mechanism supporting people’s ability to generalize from their past experience to new situations. I worked to extend and develop formal models of this mechanism, describing the way people learn to pay attention to different features of stimuli, and explaining how this attention can give rise to phenomenon apparently at odds with the exemplar formalism (Kalish & Kruschke, 2000; Kalish, 2001; Kalish, Lewandowsky & Davies, 2005).
My interest in category learning continues, with my current work dealing mainly with questions of ‘systems’ in categorization (Newell, Dunn & Kalish, 2010). The issue of multiple systems in cognition in general and category learning in particular is central to the goal of understanding cognition and its neural foundations. Vast amounts of research money and time are spent on attempts to identify the neural correlates of different systems, yet the inferred existence of these systems often hinges on what may be false dissociations in behavioral data. My aim in this research project is to employ new empirical, statistical and modeling techniques to determine the number and nature of processes underlying human category learning. On the one hand I am using State Trace Analysis, a tool for assessing the differential influence of experimental factors on behavioral outcomes with minimal theoretical commitments, to measure the dimensionality of the system supporting categorization. On the other hand I am using Hierarchical Bayesian Modeling to measure the plausibility with which different models, or mixtures of models, account for this dimensionality. The outcome will be a principled alternative model to the current dominant approach, which will not only impact theories of category learning but, by extension, the many aspects of cognition for which multiple-system explanations have been invoked.
This project represents both established and new directions in my quantitative research. My ongoing interest in category learning models is married with my emerging interest in developing new experimental methods and analytic techniques for assessing structural models.
Clarifying the mechanisms underlying function learning
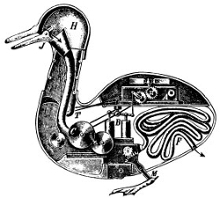
Function learning is the acquisition of knowledge of the relationship between two continuous variables. Functions are of interest because of their broad generality; many kinds of complex causal knowledge in different expert domains can be conceived of as functions. The learning of function concepts was long held to be accomplished either by tuning a parametric function or by simple generalization from stored instances. However, as Kalish, Lewandowsky & Kruschke (2004) showed, neither of these approaches is sufficient to account for a variety of results, most prominently those inspired by our earlier work (Lewandowsky, Kalish & Ngang, 2002). The account developed for these earlier findings led to novel and testable predictions, which turned out to be true. I showed that no other theory was capable of describing these results, leading to a healthy debate in the field about the representational foundations of function learning. The theory I developed is a natural outgrowth of theoretical advances in category learning, and holds that people use exemplar memory to gate access to one of many parcels of knowledge of simple relations. This theory supposes that error in prediction is used to adjust this gate, via a process analogous to the shift in dimensional attention that accompanies skill acquisition. I am continuing to advance this theory, most recently with an effort to reformulate the model in probabilistic terms – an effort that will align the model with a Bayesian computational approach (Griffiths, Lucas, Williams & Kalish, 2009), and clarify individual differences in function learning.
Clarifying the mechanisms underlying function learning
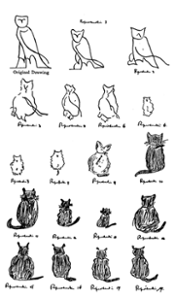
My involvement in computational approaches has led to the most recent of my research interests, an understanding of the consequences of iterated learning with Bayesian agents. Iterated learning is a situation in which an individual acquires knowledge through experience, and is then called upon to generalize that knowledge to novel situations. The results of this generalization are then provided to another individual as training data, and that individual in turn passes their knowledge on to the next. This is analogous to the transmission of knowledge across generations as formalized in studies of cultural and language evolution. In collaboration (Griffiths & Kalish, 2007) I showed that this formalism has clear and unexpected consequences for the knowledge being transmitted; namely, that in the limit the probability that any one individual will hold any given representation is completely predicted by the prior probability of that representation of the task. This means that cultural transmission reveals our inductive priors. This has two implications. First, for the study of learning (of categories, or functions) we can use a paradigm based on iterated learning to discover what the inductive priors of learners are. I have demonstrated the applicability of this in both the category and function domains. This paradigm is likely to have significant value going forward as researchers add it to their toolkit of methods for assessing prior belief. Indeed, other researchers have already used the method to explore domains such as multi-dimensional categories and color vocabulary. My own research plan includes the use of this paradigm in the study of simple concepts and linguistic structures.